Have you ever queried a generative AI, like OpenAI’s GPT or Perplexity, to describe an image? I recently asked OpenAI to describe a photograph from a U.S. presidential inauguration, and here’s what it produced:
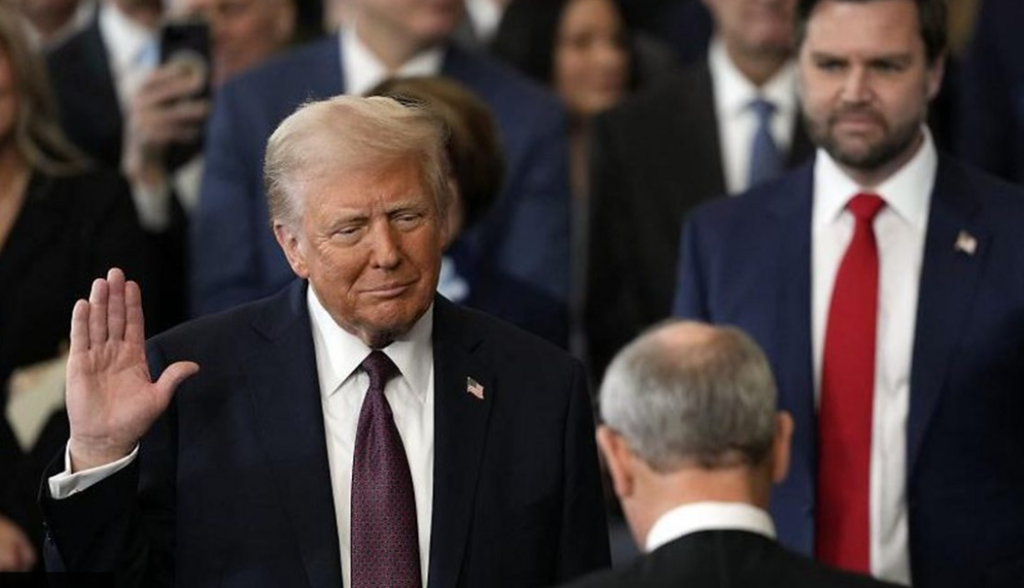
The photograph shows a formal event where a man in a dark suit, white shirt, and maroon tie is raising his right hand, as if taking an oath. He is standing in front of another man, who appears to be administering the oath. The scene is taking place in a crowded room filled with people in formal attire, suggesting this is a significant public or political ceremony.
While accurate, the response lacked essential context. It missed out on the significance of the moment and the key figures involved. In contrast, when I asked for a description of a famous painting depicting Napoleon’s Coronation, the AI hit the mark perfectly:
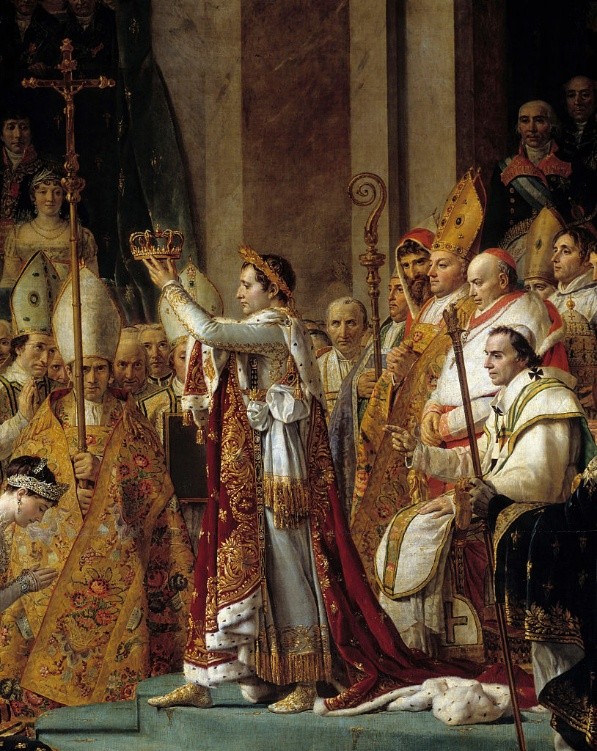
The image you’ve shared is a section of the famous painting “The Coronation of Napoleon” by Jacques-Louis David, completed in 1807. This neoclassical masterpiece captures the grandiose moment of Napoleon Bonaparte crowning himself Emperor of the French in Notre-Dame Cathedral in Paris on December 2, 1804.
So, why the difference? It all boils down to the training data available to these AI models. Due to time and cost limitations, large language models (LLMs) aren’t frequently updated. Their understanding of language and other data is fixed during training, meaning that events from just days or weeks ago are often beyond their grasp. While techniques like Retrieval Augmented Generation (RAG) help incorporate current information, without context or background details, LLMs can only describe what’s visually apparent in a photograph.
The Literal Nature of AI
There’s no denying the revolutionary impact of generative AI tools like OpenAI and Perplexity on content creation. Tasks that once required extensive time and effort can now be executed in mere minutes. However, despite their impressive functionality, these tools struggle with one crucial aspect: their literalism. Without context, they can only convey what’s in the data they’ve been trained on—nothing more.
At their core, generative AI models sift through vast data sets to identify patterns and produce coherent outputs. However, they cannot inherently infer deeper meanings or grasp cultural contexts. For instance, when tasked with describing a photograph of a presidential inauguration, the AI might detail colors and scenes meticulously, but it will completely miss the historical and emotional weight behind the image.
The Challenge of Understanding Satire
Satire relies heavily on irony, humor, and exaggeration to critique societal issues. Recognizing satire involves a nuanced understanding of context, cultural references, and underlying messages. While generative AI can mirror the structure and tone of satirical writing, it simply cannot capture the essence that makes satire resonate.
This limitation also extends to AI’s broader challenges with contextual comprehension. While the models can analyze and generate well-structured text, they often fail to appreciate the subtleties of human communication. For example, AI might produce sleek advertisements when crafting marketing content but struggle to convey a brand’s unique personality or respond to cultural nuances. This underscores the critical need for human oversight in AI-generated materials.
Why This Matters for Marketers
As consumers increasingly rely on AI-driven tools like ChatGPT and Perplexity for information, brands need to adapt. To get your products and services recognized by these LLMs in search results, focusing on creating literal, straightforward content is essential. Understanding how generative AI operates will enable your marketing strategies to gain greater visibility and engagement in an increasingly AI-dominated landscape.
Ensuring your message is clear and direct in a world where context matters can make all the difference.